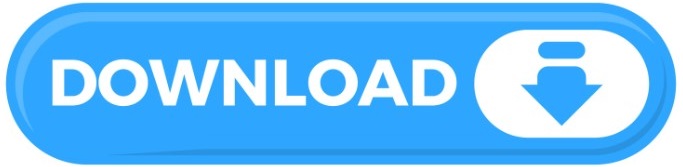
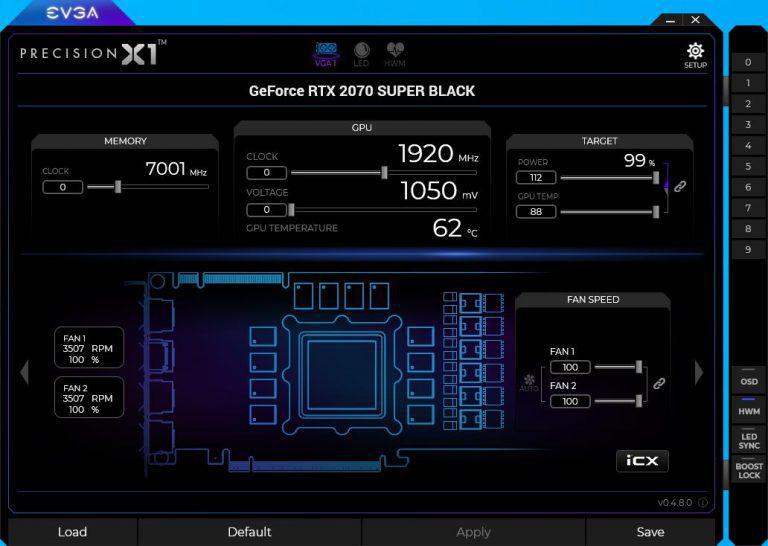
We first study how a standard 28 nm CMOS process performs in the context of deep learning accelerators design, giving special consideration to the power and area of circuits based on standard cells when reduced precision arithmetic and short SRAM memory words are used. In this thesis, we propose a set of innovations and tech- nologies belonging to one of the many research lines sparkled by such demand, focusing on energy-efficient hardware for convolutional neural networks. The achieved increase in accuracy created the demand for faster, more power-efficient hardware suited for deployment on edge devices. The growth in popularity of these algorithms has its root in the exponential increase of com- puting power available for their training consequent to the diffusion of GPUs. This option may be re-enabled by the project by placing a file with the name ".Over the last ten years, the rise of deep learning has redefined the state-of-the-art in many com- puter vision and natural language processing tasks, with applications ranging from automated personal assistants and social network filtering to self-driving cars and drug development. NOTE: As of directory index display has been disabled by default. Contact the project administrators of this project via email (see the upper right-hand corner of the Project Summary page for their usernames) at you are a maintainer of this web content, please refer to the Site Documentation regarding web services for further assistance.Contact the project via their designated support resources.If this is a severe or recurring/persistent problem, please do one of the following, and provide the error text (numbered 1 through 7, above): This issue should be reported to the -hosted project (not to ).

Reporting this problem: The problem you have encountered is with a project web site hosted by. URL path: /dl/changelog/5.4/ChangeLog-5.4. An error has been encountered in accessing this page.Ģ.
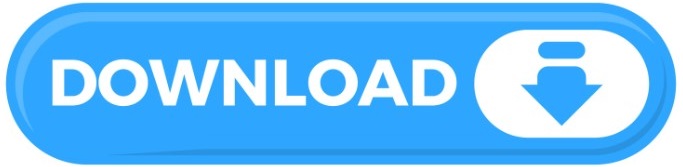